前回はRandom Forestで分類モデルを作成しました。
精度はデフォルト設定のままだったのか、0.73205でした。
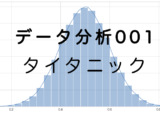
暫定1位はロジスティック回帰CVで作成してモデルで、Kaggleの精度は0.76794です。
今回はお待ちかねのXGBoostを試してみようと思います。
よくコンペで利用されるアルゴリズムですね。
XGBoost is an optimized distributed gradient boosting library designed to be highly efficient, flexible and portable. 引用: https://xgboost.readthedocs.io/en/stable/
XGBoostは最適化された分散型勾配ブースティングで高効率かつ柔軟でポータブルになるよう設計されたライブラリのようです。
ここでいうポータブルはどういう意味なんでしょうね。おそらく軽量・移植可能・独立したパッケージという意味で使われているのかも知れません。
勾配ブースティングとは下記の意味のようです。
弱い予測モデル weak prediction model(通常は決定木)のアンサンブルの形で予測モデルを生成する:https://ja.wikipedia.org/wiki/勾配ブースティング
それでは試してみます。XGBoost回帰(XGBRegressor)ではなくXGBoost分類(XGBClassifier)を使います。
評価指標
タイタニックのデータセットは生存有無を正確に予測できた乗客の割合(Accuracy)を評価指標としています。
分析用データの準備
事前に欠損値処理や特徴量エンジニアリングを実施してデータをエクスポートしています。
本記事と同じ結果にするためには事前に下記記事を確認してデータを用意してください。
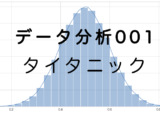
学習データと評価データの読み込み
import pandas as pd
import numpy as np
# タイタニックデータセットの学習用データと評価用データの読み込み
df_train = pd.read_csv("/Users/hinomaruc/Desktop/notebooks/titanic/titanic_train.csv")
df_eval = pd.read_csv("/Users/hinomaruc/Desktop/notebooks/titanic/titanic_eval.csv")
概要確認
# 概要確認
df_train.info()
RangeIndex: 891 entries, 0 to 890 Data columns (total 22 columns): # Column Non-Null Count Dtype --- ------ -------------- ----- 0 PassengerId 891 non-null int64 1 Survived 891 non-null int64 2 Pclass 891 non-null int64 3 Name 891 non-null object 4 Sex 891 non-null object 5 Age 891 non-null float64 6 SibSp 891 non-null int64 7 Parch 891 non-null int64 8 Ticket 891 non-null object 9 Fare 891 non-null float64 10 Cabin 204 non-null object 11 Embarked 891 non-null object 12 FamilyCnt 891 non-null int64 13 SameTicketCnt 891 non-null int64 14 Pclass_str_1 891 non-null float64 15 Pclass_str_2 891 non-null float64 16 Pclass_str_3 891 non-null float64 17 Sex_female 891 non-null float64 18 Sex_male 891 non-null float64 19 Embarked_C 891 non-null float64 20 Embarked_Q 891 non-null float64 21 Embarked_S 891 non-null float64 dtypes: float64(10), int64(7), object(5) memory usage: 153.3+ KB
# 概要確認
df_eval.info()
RangeIndex: 418 entries, 0 to 417 Data columns (total 21 columns): # Column Non-Null Count Dtype --- ------ -------------- ----- 0 PassengerId 418 non-null int64 1 Pclass 418 non-null int64 2 Name 418 non-null object 3 Sex 418 non-null object 4 Age 418 non-null float64 5 SibSp 418 non-null int64 6 Parch 418 non-null int64 7 Ticket 418 non-null object 8 Fare 418 non-null float64 9 Cabin 91 non-null object 10 Embarked 418 non-null object 11 Pclass_str_1 418 non-null float64 12 Pclass_str_2 418 non-null float64 13 Pclass_str_3 418 non-null float64 14 Sex_female 418 non-null float64 15 Sex_male 418 non-null float64 16 Embarked_C 418 non-null float64 17 Embarked_Q 418 non-null float64 18 Embarked_S 418 non-null float64 19 FamilyCnt 418 non-null int64 20 SameTicketCnt 418 non-null int64 dtypes: float64(10), int64(6), object(5) memory usage: 68.7+ KB
# 描画設定
import seaborn as sns
from matplotlib import ticker
import matplotlib.pyplot as plt
sns.set_style("whitegrid")
from matplotlib import rcParams
rcParams['font.family'] = 'Hiragino Sans' # Macの場合
#rcParams['font.family'] = 'Meiryo' # Windowsの場合
#rcParams['font.family'] = 'VL PGothic' # Linuxの場合
rcParams['xtick.labelsize'] = 12 # x軸のラベルのフォントサイズ
rcParams['ytick.labelsize'] = 12 # y軸のラベルのフォントサイズ
rcParams['axes.labelsize'] = 18 # ラベルのフォントとサイズ
rcParams['figure.figsize'] = 18,8 # 画像サイズの変更(inch)
モデリング用に学習用データを訓練データとテストデータに分割
# 訓練データとテストデータに分割する。
from sklearn.model_selection import train_test_split
x_train, x_test = train_test_split(df_train, test_size=0.20,random_state=100)
# 説明変数
FEATURE_COLS=[
'Age'
, 'Fare'
, 'SameTicketCnt'
, 'Pclass_str_1'
, 'Pclass_str_3'
, 'Sex_female'
, 'Embarked_Q'
, 'Embarked_S'
]
X_train = x_train[FEATURE_COLS] # 説明変数 (train)
Y_train = x_train["Survived"] # 目的変数 (train)
X_test = x_test[FEATURE_COLS] # 説明変数 (test)
Y_test = x_test["Survived"] # 目的変数 (test)
XGBoost (デフォルト設定)
# https://xgboost.readthedocs.io/en/stable/parameter.html
# https://xgboost.readthedocs.io/en/stable/python/python_api.html?#xgboost.XGBClassifier
import xgboost as xgb
clf = xgb.XGBClassifier(random_state=10,verbosity=1,use_label_encoder=False)
warningがでるので、use_label_encoder=Falseを設定しています。
モデル作成
# fitで学習させる
clf.fit(X_train,Y_train)
[08:29:20] WARNING: /private/var/folders/42/7v1wzyk12qd5_mvhxl3r6r9h0000gn/T/pip-install-oa2np12c/xgboost_2e2a52630a8a4d8288ffb85fdfa9ef34/build/temp.macosx-10.13-x86_64-3.9/xgboost/src/learner.cc:1115: Starting in XGBoost 1.3.0, the default evaluation metric used with the objective 'binary:logistic' was changed from 'error' to 'logloss'. Explicitly set eval_metric if you'd like to restore the old behavior. XGBClassifier(base_score=0.5, booster='gbtree', colsample_bylevel=1, colsample_bynode=1, colsample_bytree=1, enable_categorical=False, gamma=0, gpu_id=-1, importance_type=None, interaction_constraints='', learning_rate=0.300000012, max_delta_step=0, max_depth=6, min_child_weight=1, missing=nan, monotone_constraints='()', n_estimators=100, n_jobs=1, num_parallel_tree=1, predictor='auto', random_state=10, reg_alpha=0, reg_lambda=1, scale_pos_weight=1, subsample=1, tree_method='exact', use_label_encoder=False, validate_parameters=1, verbosity=1)
精度確認
# Return the mean accuracy on the given data and labels.
print("train",clf.score(X_train,Y_train))
print("test",clf.score(X_test,Y_test))
train 0.9775280898876404 test 0.7821229050279329
clf.get_params()
{'objective': 'binary:logistic', 'use_label_encoder': False, 'base_score': 0.5, 'booster': 'gbtree', 'colsample_bylevel': 1, 'colsample_bynode': 1, 'colsample_bytree': 1, 'enable_categorical': False, 'gamma': 0, 'gpu_id': -1, 'importance_type': None, 'interaction_constraints': '', 'learning_rate': 0.300000012, 'max_delta_step': 0, 'max_depth': 6, 'min_child_weight': 1, 'missing': nan, 'monotone_constraints': '()', 'n_estimators': 100, 'n_jobs': 1, 'num_parallel_tree': 1, 'predictor': 'auto', 'random_state': 10, 'reg_alpha': 0, 'reg_lambda': 1, 'scale_pos_weight': 1, 'subsample': 1, 'tree_method': 'exact', 'validate_parameters': 1, 'verbosity': 1}
xgb.plot_importance(clf)
<AxesSubplot:title={'center':'Feature importance'}, xlabel='F score', ylabel='Features'>
# https://scikit-learn.org/stable/modules/generated/sklearn.metrics.ConfusionMatrixDisplay.html
import matplotlib.pyplot as plt
from sklearn.datasets import make_classification
from sklearn.metrics import ConfusionMatrixDisplay
from sklearn.metrics import confusion_matrix
print(confusion_matrix(Y_test,clf.predict(X_test)))
ConfusionMatrixDisplay.from_estimator(clf,X_test,Y_test,cmap="Reds",display_labels=["非生存","生存"],normalize="all")
plt.show()
[[84 20]
[19 56]]
Kaggleへ予測データをアップロード
df_eval["Survived"] = clf.predict(df_eval[FEATURE_COLS])
df_eval[["PassengerId","Survived"]].to_csv("titanic_submission.csv",index=False)
!/Users/hinomaruc/Desktop/notebooks/my-venv/bin/kaggle competitions submit -c titanic -f titanic_submission.csv -m "model #008. xgboost"
100%|████████████████████████████████████████| 2.77k/2.77k [00:05<00:00, 555B/s] Successfully submitted to Titanic - Machine Learning from Disaster
0.74880
RandomForestよりはいいけど、、という感じですね。
デフォルトの設定ではなく、GridSearchでパラメーターをチューニングしてみようと思います。
XGBoost (GridSearchでチューニング)
# https://xgboost.readthedocs.io/en/stable/parameter.html
# https://xgboost.readthedocs.io/en/stable/python/python_api.html?#xgboost.XGBClassifier
import xgboost as xgb
from sklearn.metrics import mean_absolute_error
from sklearn.model_selection import GridSearchCV
from sklearn.model_selection import KFold
# Grid Search用のパラメータ作成。
# あまり組み合わせが多いと時間がかかる
params = {
'eta': [0.1,0.3,0.6], # default = 0.3
'gamma': [0,1,2], # default = 0
'max_depth': [2,4,6], # default = 6
'min_child_weight': [0,1,2], # default = 1
'subsample': [0.8,0.9,1], # default = 1
'colsample_bytree': [0,0.5,1], # default = 1
}
# K-分割交差検証
kf = KFold(n_splits = 5, shuffle = True, random_state = 1)
# モデル定義
clf = xgb.XGBClassifier(random_state=10,verbosity=1,use_label_encoder=False)
# Gridサーチ定義
# https://scikit-learn.org/stable/modules/model_evaluation.html#common-cases-predefined-values
grid = GridSearchCV(estimator=clf, param_grid=params, scoring='balanced_accuracy', n_jobs=2, cv=kf.split(X_train,Y_train), verbose=1)
scoringは色々な指標があるようです。今回はbalanced_accuracyを選択してみました。各指標についてはもう少し勉強して記事にまとめようと思います。
モデル作成
# fitで学習させる
grid.fit(X_train,Y_train)
Fitting 5 folds for each of 729 candidates, totalling 3645 fits in XGBoost 1.3.0, the default evaluation metric used with the objective 'binary:logistic' was changed from 'error' to 'logloss'. Explicitly set eval_metric if you'd like to restore the old behavior. ・・・省略・・・ GridSearchCV(cv=, estimator=XGBClassifier(base_score=None, booster=None, colsample_bylevel=None, colsample_bynode=None, colsample_bytree=None, enable_categorical=False, gamma=None, gpu_id=None, importance_type=None, interaction_constraints=None, learning_rate=None, max_delta_step=None, max_depth=None, min_child_weigh... random_state=10, reg_alpha=None, reg_lambda=None, scale_pos_weight=None, subsample=None, tree_method=None, use_label_encoder=False, validate_parameters=None, verbosity=1), n_jobs=2, param_grid={'colsample_bytree': [0, 0.5, 1], 'eta': [0.1, 0.3, 0.6], 'gamma': [0, 1, 2], 'max_depth': [2, 4, 6], 'min_child_weight': [0, 1, 2], 'subsample': [0.8, 0.9, 1]}, scoring='balanced_accuracy', verbose=1)
# 学習結果確認
print('ベストスコア:',grid.best_score_, sep="\n")
print('\n')
print('ベストestimator:',grid.best_estimator_,sep="\n")
print('\n')
print('ベストparams:',grid.best_params_,sep="\n")
ベストスコア: 0.810071880374643 ベストestimator: XGBClassifier(base_score=0.5, booster='gbtree', colsample_bylevel=1, colsample_bynode=1, colsample_bytree=0.5, enable_categorical=False, eta=0.6, gamma=1, gpu_id=-1, importance_type=None, interaction_constraints='', learning_rate=0.600000024, max_delta_step=0, max_depth=2, min_child_weight=1, missing=nan, monotone_constraints='()', n_estimators=100, n_jobs=1, num_parallel_tree=1, predictor='auto', random_state=10, reg_alpha=0, reg_lambda=1, scale_pos_weight=1, subsample=1, tree_method='exact', use_label_encoder=False, validate_parameters=1, verbosity=1) ベストparams: {'colsample_bytree': 0.5, 'eta': 0.6, 'gamma': 1, 'max_depth': 2, 'min_child_weight': 1, 'subsample': 1}
精度確認
# Grid Searchで一番精度が良かったモデル
best_clf = grid.best_estimator_
# Return the mean accuracy on the given data and labels.
print("train",best_clf.score(X_train,Y_train))
print("test",best_clf.score(X_test,Y_test))
train 0.8735955056179775 test 0.8435754189944135
過学習は抑えられたようです。
# モデルパラメータ一覧
best_clf.get_params()
{'objective': 'binary:logistic', 'use_label_encoder': False, 'base_score': 0.5, 'booster': 'gbtree', 'colsample_bylevel': 1, 'colsample_bynode': 1, 'colsample_bytree': 0.5, 'enable_categorical': False, 'gamma': 1, 'gpu_id': -1, 'importance_type': None, 'interaction_constraints': '', 'learning_rate': 0.600000024, 'max_delta_step': 0, 'max_depth': 2, 'min_child_weight': 1, 'missing': nan, 'monotone_constraints': '()', 'n_estimators': 100, 'n_jobs': 1, 'num_parallel_tree': 1, 'predictor': 'auto', 'random_state': 10, 'reg_alpha': 0, 'reg_lambda': 1, 'scale_pos_weight': 1, 'subsample': 1, 'tree_method': 'exact', 'validate_parameters': 1, 'verbosity': 1, 'eta': 0.6}
xgb.plot_importance(best_clf)
<AxesSubplot:title={'center':'Feature importance'}, xlabel='F score', ylabel='Features'>
# https://scikit-learn.org/stable/modules/generated/sklearn.metrics.ConfusionMatrixDisplay.html
import matplotlib.pyplot as plt
from sklearn.datasets import make_classification
from sklearn.metrics import ConfusionMatrixDisplay
from sklearn.metrics import confusion_matrix
print(confusion_matrix(Y_test,best_clf.predict(X_test)))
ConfusionMatrixDisplay.from_estimator(best_clf,X_test,Y_test,cmap="Reds",display_labels=["非生存","生存"],normalize="all")
plt.show()
[[96 8]
[20 55]]
ロジスティック回帰CVの結果は下記なので、それよりはいい結果になっているようです。
[[95 9]
[27 48]]
あとは、実際にKaggleにアップしたときの精度がどうなっているか確認してみます。
Kaggleへ予測データをアップロード
df_eval["Survived"] = best_clf.predict(df_eval[FEATURE_COLS])
df_eval[["PassengerId","Survived"]].to_csv("titanic_submission.csv",index=False)
!/Users/hinomaruc/Desktop/notebooks/my-venv/bin/kaggle competitions submit -c titanic -f titanic_submission.csv -m "model #008. xgboost grid search"
100%|████████████████████████████████████████| 2.77k/2.77k [00:04<00:00, 578B/s] Successfully submitted to Titanic - Machine Learning from Disaster
0.76076
GridSearchを使わない場合より、精度が向上しました。
ただ、ロジスティック回帰CVの精度は0.76794だったので暫定1位の座は奪えませんでした。
まとめ
XGBoostだと0.8は超えるかなと思っていたので、今回作ったモデリング用データだとここら辺の数値が限界なのかもしれません。(それともまだ過学習気味なのか? 訓練データの学習ではテストデータでは表現できていない人物がいるのかもしれません。)
もうちょっと特徴量エンジニアリングを頑張ってみるなど検討の余地はありそうです。
次回はautomlを試してみようと思います。